Client Case Study
In the highly competitive telecom sector, broadband operators have long been battling for market share while grappling with declining profit margins. This intense rivalry has made customer retention a strategic imperative for ISPs.
Retaining existing customers is significantly more cost-effective than acquiring new ones, which requires substantial investments in marketing and promotions. Additionally, loyal customers are much more effective in driving revenue growth, as they are more likely to purchase additional services compared to newly acquired ones.[1]
While price remains a critical factor for customers selecting a broadband package, the ability of an ISP to deliver an exceptional customer experience (CX) and industry-leading support is also a crucial source of competitive advantage[2]. Machine learning is a powerful tool for enhancing customer experience and reducing churn. Using predictive models to analyze past customer behavior can identify early warning signs of churn and high-risk segments, enable proactive support and interventions, and guide better product development to improve retention.
Here’s how Cartesian used machine learning to develop a series of predictive models that helped our client enhance their customer experience and improve their customer retention strategies to get ahead of churn.
How We Used Machine Learning & AI to Predict & Reduce Churn
A leading ISP in the UK partnered with Cartesian to tackle customer churn in their broadband offerings. Cartesian used our proven data science and machine learning expertise to build a holistic customer experience (CX) model, which our client used to improve product development and boost user satisfaction and customer retention rates.
Building the Churn Prediction Models
Our methodology involved several key steps to develop a suite of custom machine learning (ML) models aimed at predicting our client’s user churn.
Here’s how we did it:
- Data Preparation – Cartesian built an extensive data pipeline to collect and aggregate data across multiple sources:
- a. Product performance metrics: This included technical KPIs on routers and extenders, such as characteristics and quality of service indicators. For example, we analyzed line speed, signal strength, and levels of in-house interference to understand how these factors impact user experience.
- b. User behavior: We collected data on users’ line usage, including download and upload traffic. Additionally, we examined household characteristics, such as the number of connected devices, to see how these factors correlated with churn.
- c. Customer profile: This involved gathering information on the products purchased by customers and their demographics, such as age, location, and service tenure. Understanding and applying customer segmentation to these profiles helped us identify patterns and trends related to churn in key user segments.
- Predictive Modeling
- a. Feature engineering: This step involved transforming raw data points into meaningful figures that could be used in our churn prediction models. We cleaned the data by examining data distributions to detect and exclude outliers and address anomalies, ensuring the data was robust and generalizable.
- b. Model development: We trained various classification algorithms, including decision trees, random forests, and gradient boosting machines to develop churn prediction models. We evaluated these models based on their accuracy, precision, recall, and other relevant metrics. The goal was to select the algorithms with the highest predictability for identifying customers at high risk of churning.
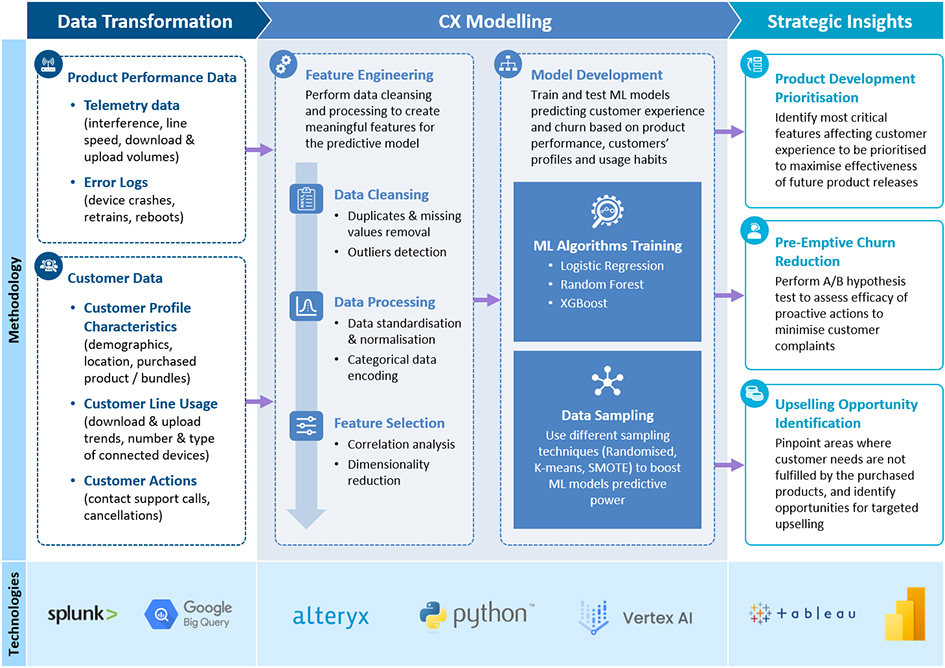
By matching router performance data to customer profile characteristics and usage habits, the models provided a roadmap for future product developments and informed effective retention strategies to minimize churn.
Our Predictive Churn Models in Action
Cartesian’s predictive models have been instrumental in providing our client with strategic and actionable insights for their churn reduction initiatives in the following key areas:
- Pre-emptive Churn Reduction: We conducted A/B testing to evaluate the effectiveness of proactive measures in mitigating the impact of service disruptions on the customer experience of customers with high churn risk.
- Identifying Localized Issues: We identified clusters of customers in specific locations who were more exposed to network issues and therefore more likely to churn. This allowed the client to target these high-value, high-problem areas for network optimizations to improve overall customer satisfaction.
- Product Development Prioritization: Some quality-of-service KPIs are more highly correlated with churn than others, and product development should be prioritized in such a way that future releases take them into consideration to maximize customer retention. We provided our client with a prioritized roadmap of the most important new features to focus on first.
- Recognizing Upsell Opportunities: By analyzing customer personas derived from usage habits and profiles, the predictive model identified mismatches with current offerings, uncovering potential upselling opportunities.
Harness the Power of Machine Learning & Predictive Modeling with Cartesian
Cartesian’s staff of telecom and data science experts have decades of collective experience building predictive machine learning models and applying them across a wide range of problems and initiatives. Contact Cartesian today to learn more about how we can partner with your organization.